
Date: Tue Jun 26, 2018
Time: 1:15 PM - 3:00 PM
Moderator: Asim Biswas
The objective of this research was to develop an active crop sensor-based precision rice (Oryza sativa L.) management (PRM) strategy to improve rice yield, N and water use efficiencies and evaluate it against farmer’s rice management in Northeast China. Two field experiments were conducted from 2011 to 2013 in Jiansanjiang, Heilongjiang Province, China, involving four treatments and two varieties (Kongyu 131 and Longjing 21). The results indicated that PRM system significantly increased rice grain yield, N recovery efficiency (RE), agronomic efficiency (AE) and partial factor productivity (PFP) by an average of 12%, 63%, 89% and 53% over FP system across two varieties and the three years, respectively. Water use efficiency was increased by 59-60%. It is concluded that the PRM system can significantly increase rice yield, N and water use efficiencies than farmer’s practices and has the potential to contribute to both food security and sustainable development.
Cereal rye (cereale secale L.) is a winter crop well suited for cultivation especially besides high yield areas because of its relatively low demands on the soil and on the climate as well. In 2016 about 4.9% of arable land in Germany was cultivated with cereal rye (Statistisches Bundesamt, 2017).
Unlike other crops such as wheat, there is little research on cereal rye for site specific farming. Furthermore, also in a cereal rye cultivation it is necessary to minimize nitrogen loss. This is especially important in low yield areas to avoid any lodging of the plants because of an overdose of mineral nitrogen (N). Therefore, an efficient fertilization strategy becomes important. To achieve this goal, some essential crop parameters such as above-ground plant biomass, N-content and N-uptake are necessary. The objective of this work was to evaluate the suitability of various vegetation indices to describe the N-uptake of cereal rye.
Over a period of nine years, from 2007 to 2016 two different kinds of plot experiments were conducted. The first one was a fertilization experiment, which consisted of only one variety of cereal rye treated with nine different N-rates from 0 up to 250 kg N per hectare (ha) which were applied at different growth stages. The second experiment consisted of four different varieties (population varieties as well as hybrids) and was treated with two different N-rates (100 and 160 kg N per ha). Each of the two experiments was designed as a double plot with four replications, in which one was for non-conducting reflectance measurements of the canopy during the vegetation period, and at the end harvesting with a plot combine; whereas the neighboring plot was for biomass sampling. Biomass samples for nitrogen analysis and sensor measurements have been performed four times during the growing season at certain growth stages.
The results indicated that certain vegetation indices (VI) calculated from reflectance measurements represented well the N-uptake of the crops at different growth stages. Especially simple ratios, calculated from two different wavelengths, as well as the Red Edge Inflection Point (REIP) represent best the N-uptake and the influence of the different cultivars was negligible. In comparison, the coefficients of determination of the widely known NDVI were only good at an early growth stage and, in addition, the higher the N-uptake was, the more NDVI showed a saturation effect at later growth stages. By using the NDVI-formula with other spectral areas to describe crop parameters the results were different. The saturation effect almost disappeared and the coefficient of determination increased significantly.
Crop yield estimation and mapping are important tools that can help growers efficiently use their available resources and have access to detailed representations of their farm. Technical advancements in computer vision have improved the detection, quality assessment and yield estimation processes for crops, including apples, citrus, mangoes, maize, figs and many other fruits. However, similar methods capable of exporting a detailed yield map for vegetable crops have not yet been fully developed. A machine vision-based yield monitor was designed to perform identification and continuous counting of shallot onions in-situ during the harvesting process. The system is composed of a video and position logger, coupled with acomputer software, and can be used within the tractor itself. A modular camera bracket collected video data of the crops while positioned directly above the harvesting conveyor. Video data was collected in real-time with natural sunlight conditions and in a semi-controlled lighting environment using an artificial light source to enhance vegetable areas. Computational analysis was performed to track detected vegetables on the conveyor. The system is to be tested for a full continuous run during the summer 2018 harvesting season. Based on preliminary results, occasional occlusion of vegetables and inconsistent light conditions are the main limiting factors that may inhibit performance. Although further enhancements are envisioned for the prototype system developed, it has the potential to benefit many producers of small vegetable crops by providing them with useful harvest information in real time and can help to improve harvesting logistics.
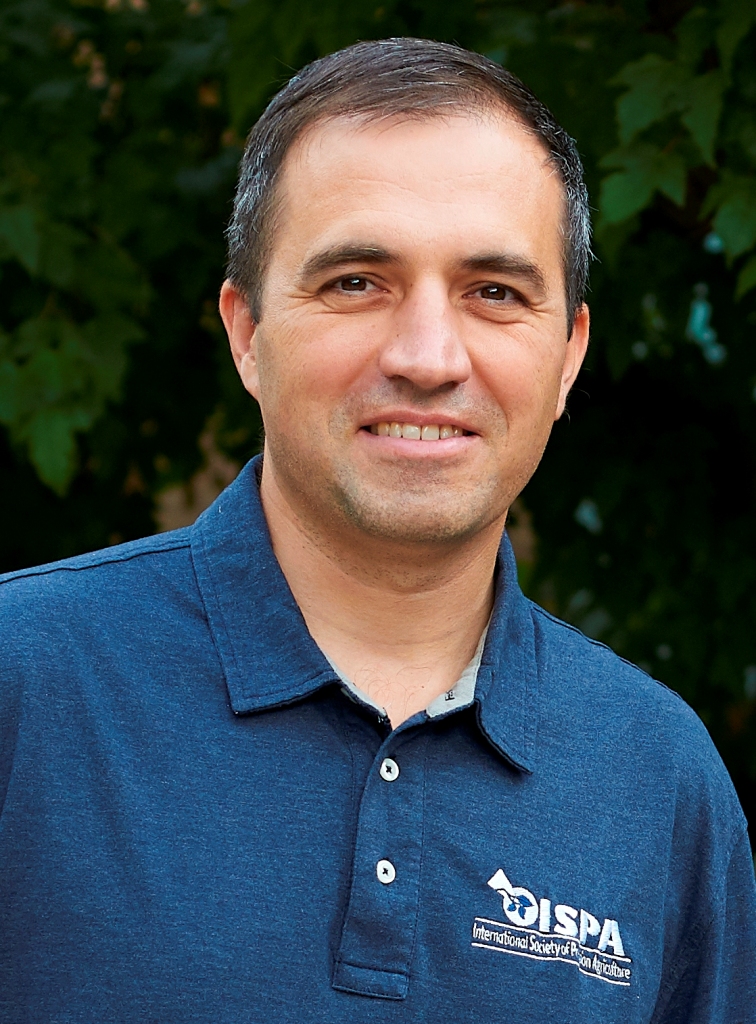
Yield monitoring of potato in real time during harvesting would be useful for farmers, providing instant yield and income information. In the study, potentials of candidate sensors were evaluated with different yield measurement techniques for yield monitoring system of small-sized potato harvesters. Mass-based (i.e., load cell) and volume-based (i.e., CCD camera) sensors were selected and tested under laboratory conditions. For mass-based sensing, an impact plate instrumented with load cells was placed so that the potatoes discharged from the transportation part were contacted before they fell down to the collection part. Load cell signals due to the plate bending by the impact force were calibrated to the mass of the potatoes. Effects of potato dropping height on load cell was investigated for single potato and multiple potatoes. For volume-based sensing, a CCD camera was installed above the transportation part so that the top and side images of the potatoes were captured. Area and volume were obtained from the original images and calibrated to the mass of potatoes. The calibration tests of potato showed linear calibrations with R2 of 0.98 for potatoes dropped from a height of 30 cm for the mass-based and 0.37 for volume-based approaches. This study showed potentials of candidate sensors for yield monitoring of potato yield monitoring system. Further study would be necessary to investigate the effects of vibration and harvester inclination for field application.
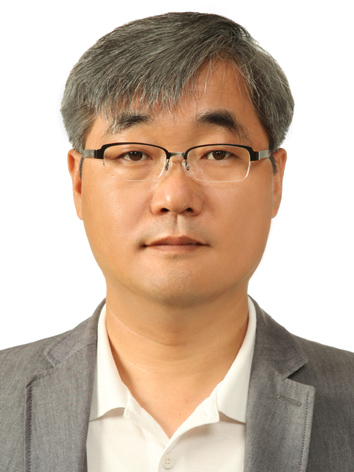
While modern grain yield monitors are able to harvest variety and hybrid trials without imposing bias, cotton yield monitors are affected by varietal properties. With planters capable of site-specific planting of multiple varieties, it is essential to better understand cotton yield monitor calibration. Large-plot field experiments were conducted with two southeast Missouri cotton producers to compare yield monitor-estimated weights and observed weights in replicated variety trials. Two replications of multiple varieties were planted in 12-row plots with 0.97 m row spacing. Plots were harvested with a module-building spindle picker equipped with a yield monitor. A separate module was built for each plot and weighed. Yield monitor data were used to calculate an estimated weight for each module. Significant differences in seed cotton yields were detected between the observed (weighed modules) and estimated (yield monitor) values. In addition to a significant variety main effect, a significant location by variety interaction was present in the error, both in terms of yield (absolute) and as a percentage of the observed yield (relative). Some HVI properties were significantly correlated with the absolute and relative error. Data from additional site-years will be analyzed and other factors will be investigated to try and achieve a better understanding of the factors affecting cotton yield monitor calibration.
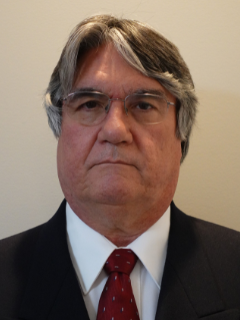
Proximal imaging using tractor-mounted cameras is a simple and cost-effective method to acquire large quantities of data in orchards and vineyards. It can be used for the monitoring of vegetation and for the management of field operations such as the guidance of smart spraying systems for instance. One of the most prolific research subjects in arboriculture is fruit detection during the growing season. Estimations of fruit-load can be used for early yield assessments and for the monitoring of harvest and thinning. In addition, the visual aspects of fruits enable to appraise their growth and ripening status. This paper proposes a new approach for real-time fruit detection, combining a fast geometrical pre-processing whose output feeds a deep neural network (DNN) classifier. The first step is a radial Hough-like operator, which aims at identifying quickly the regions of interest, restricting the use of the DNNs to the most probably genuine candidates. The proposed method is generic enough to be applied on most near-spherical fruits. It was tested in two contexts: grapes and apples, with different varieties and phenological stages. In both cases the proposed method provided promising results. Correlation coefficients with manual counting and real harvest loads are up to 0.96 for grapes and up to 0.85 for apples.
Irrigation accounts for about 80% consumptive use of water in the Northwest of United States. Even small increases in water use efficiency can improve crop production, yield, and have more water available for alternative uses. Center pivot irrigation systems are widely recognized in the irrigation industry for being one of the most efficient sprinkler systems. In recent years, there has been a shift from high pressure impact sprinklers on the top of center pivots to Mid Elevation Spray Application (MESA) sprinkler configurations and towards Low Elevation Spray Application (LESA) sprinklers. Although LESA offers range of benefits over MESA, such technologies have grower adoption concerns as the effects of these systems on the crop growth and yield are unknown. In this study, these parameters were evaluated for LESA and MESA using a small Unmanned Aerial System (UAS) integrated with multispectral and thermal imaging sensors, in corn (Zea mays var. indentata) and mint (Mentha spicata and Mentha × piperita). The field experiment was designed to have two adjacent spans of a center pivot sprinkler irrigation system with LESA and MESA in both the fields located in the state of Washington, USA. Aerial data was collected throughout the crop growing season and analyzed using image processing algorithms, custom developed in Matlab® to observe the temporal variation of the above-mentioned crop parameters for both sprinkler system configurations. Various vegetation indices and canopy temperature was extracted from the imaging data and compared for the LESA and MESA irrigated areas. Two sample T-test was performed to find if there was any significant difference at 5% level in the observed parameters between LESA and MESA.
Results showed that for mint, LESA irrigated areas had more average crop vigor and similar canopy temperature during the entire crop growth season though the difference was not significant. The LESA irrigated areas had significantly more crop vigor and less canopy temperature till the mid growth season which is the phase that determines the yield, according to many prior studies. However, for corn, MESA had more crop vigor and a cooler canopy than LESA throughout the season. Though the difference in crop vigor was not significant, the MESA irrigated canopy areas was significantly cooler than LESA irrigated areas. The results were anticipated, as the sprinkler heads used in LESA were being pulled off in corn field, causing the weighted hose to damage the corn which could be observed from the aerial images. A different kind of sprinkler head was used after this incident. However, some strips of corn had already been damaged. The damaged strips could not cause any significant difference in the canopy vigor with the MESA irrigated areas. As LESA had similar effect on canopy as MESA, LESA could be installed in mint and corn fields, backed up by several benefits of this system over MESA made in other studies, improving the water efficiency. Also, the methods developed could be used for other applications related to precision and sustainable agriculture.
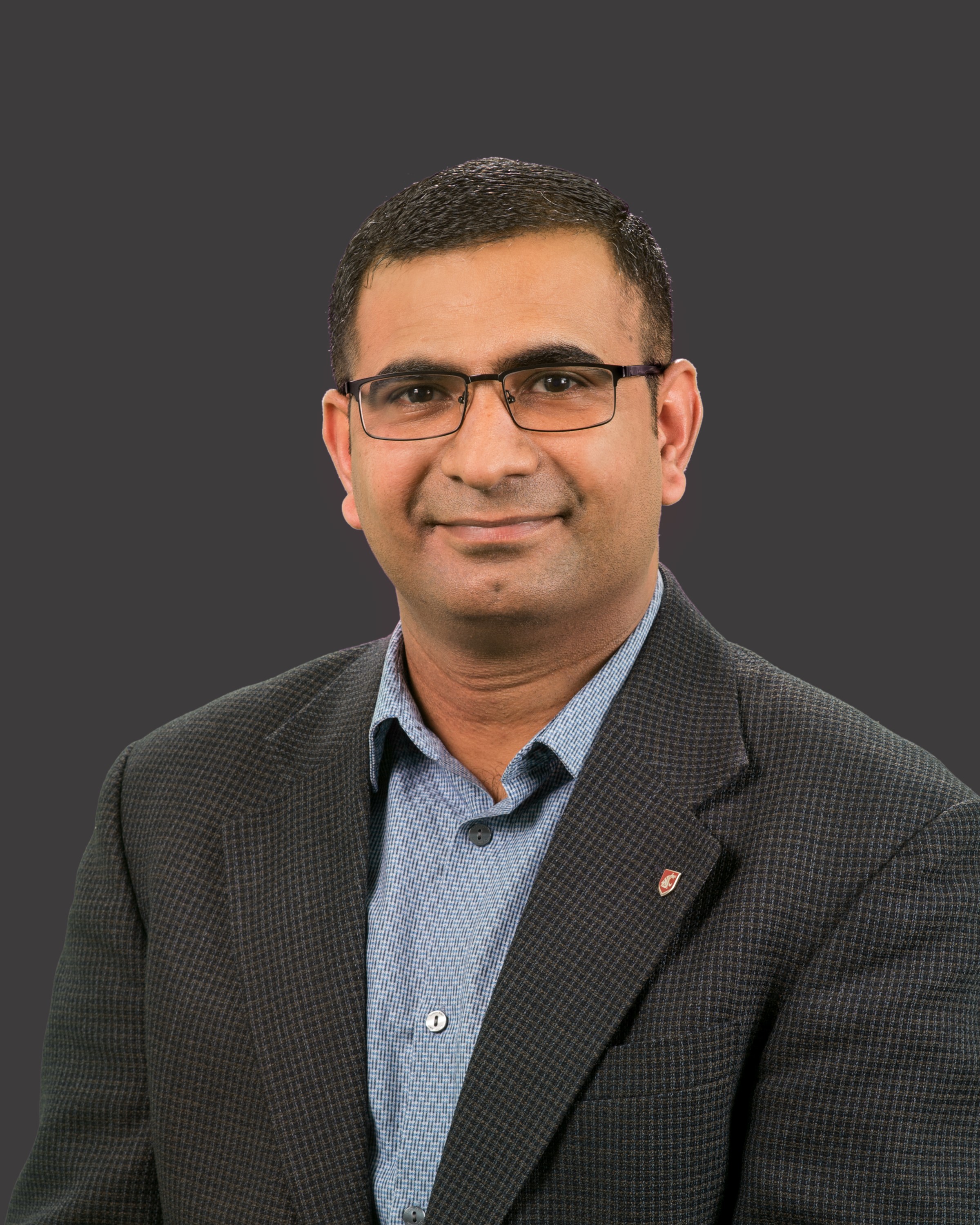